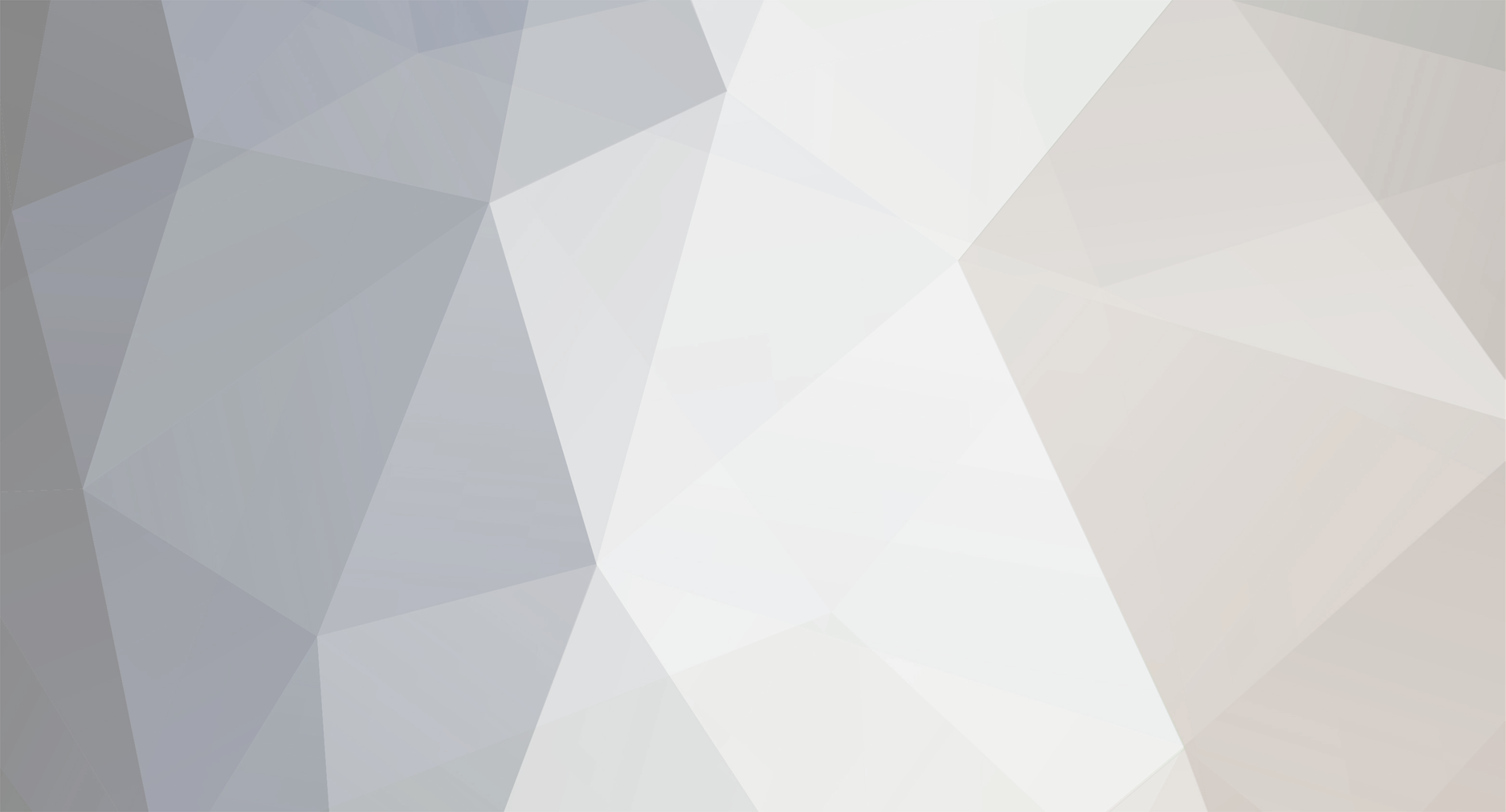
Bartholomew
Member-
Posts
642 -
Joined
-
Last visited
Content Type
Forums
Status Updates
Blogs
Events
Gallery
Downloads
Store Home
Everything posted by Bartholomew
-
Not sure... given both horizontal and vertical stripes there's also a chance it's the monitor. Some bad contact that solves when after a few minutes of warming up. Maybe try another cable and or device to see if it's the monitor or the gpu.
- 2 replies
-
- troubleshoot
- artifact
-
(and 2 more)
Tagged with:
-
Hard to say without pictures. But most likely that fan in a odd position and orientation just causes turbulence in the case instead of flow, causing more internal recirculation. Also, gpu fans suck air into the cards cooler, so any fan should blow at the card, not suck air away from the gpu's own intake fans (that would also rocket temps).
-
Yes it can. Since it's non conductive, it can prevent proper contact if in-between the cpu and the socket for one or more pins for example. To much paste "on top" of the ihs, or even splattered around the pcb is OK. But not on sockets (cpu, ram dimms) or connectors; that can prevent contact et-all, or form a resistor or even some slight capacitance etc. That can do bad / weird things to signals.
-
Might be a bad contact on the board, which could also explain it working in a different machine (the board being handled, under different tension in slot, different ambient temp, different tension from power cables). Could try what the above poster said, since it could be any component/contact, you might get lucky. To see a example of intermittent error 43 and repair:
-
I can't find my m.2 on bootable device
Bartholomew replied to Jonathan Paskalis's topic in Storage Devices
M.2 could be sata or nvme, not knowing which it is ill just list a few bios options for both, and sounds like legacy install perhaps. Note that some might be named different or n/a depending on the mobo. PCH, sata mode : AHCI Launch CSM: enable Fast boot: disable Boot device control: UEFI and legacy Boot from storage: legacy first Boot from pcie: legacy first Note that (like when uefi install) you might need diff settings but these are a few to fiddle with. Hope you get it booting again -
Tomahawk x570, only spins when needed (I actually thought this was pretty much standard for x570 boards?), which I find in 2 cases: heavy i/o on multiple ssds at once, or when ambient in the case rises significantly, like when gaming or ml learning for some time. In either case, it never bothered me, don't notice it over gpu or casefans.
- 7 replies
-
- motherboard
- fan
-
(and 3 more)
Tagged with:
-
Computer makes error code beeps then boots into windows
Bartholomew replied to mikeyk365's topic in Troubleshooting
Is it a 3000 series RTX gpu? As this is relatively common with some ryzen mobo/ 3000 rtx gpu combos. I'm on tomahawk x570 which does 4 short beeps only with my 3090, but doesn't with my old 2060. Works fine though. Some say bios update of mobo, or updated vbios helped but I never bothered as it works fine. Your case might have a different cause though -
Try to clear the drivers shadercache , especially when having changed settings a lot. Can't tell you how since that changes all the time, try to Google for it but be carefull to look at the dates of what is posted cause again: how to do it has often changed. Note that if the shader cache is cleared you'll likely notice 1st time load of game/level might take longer than usual (might depend on overall system speed though, so you might or might not notice).
-
https://www.gpufanreplacement.com/collections/gigabyte-graphics-card-fan-replacements/products/gigabyte-rtx-3060-rtx-3060ti-vision-fan-replacement-model-pld08010s12h Can also check out aliexpress, sometimes that's cheaper than the above linked site (but do consider possible import taxes)
-
It's set to private, so can't look at the suspect list
-
Hot air is coming out from exhaust while doing normal tasks
Bartholomew replied to DeadDabster's topic in Troubleshooting
This sounds like possibly a malicious process that uses 100% gpu which attempts to go unnoticed by hiding/stopping itself if taskmanager is opened. I've been on linux for while now so my knowledge on alternative ways (beyond taskman) of inspecting processes and their resource use is rusty when it comes to windows, hopefully other kind souls can advice on that (and perhaps good malware scanner). -
Yeah as the log suggests unlikely a hardware fault. Remembers me of a time where I had issues with my 2060 few years back; Nvidia drivers being "iffy". Could try going back one driver version if the issues are recent. And do note ddu itself can be "iffy" as well, haven't used it in ages but thought is should be run as administrator and required a restart (but again long time ago so don't quote me on that). Perhaps ask a mod to move the topic to graphics cards or to troubleshooting as at this time it seems unrelated to the psu; that could improve odds of getting more responses. Hope you work it out soon!
-
Red light means no or insufficient power to gpu so doing as the above poster said should resolve the issue. Edit: crossed the "already fixed" above. Congrats on OP
-
Tesla M40 24GB GPU: very poor machine-learning performance?
Bartholomew replied to rbbrdckybk's topic in Graphics Cards
Hi, The 3060 should be about 3x to 4x faster in Cuda compute performance, so expected on the m40 would be approx 12m give or take. It might depend on the model / operation structure though, if there's a lot of Cuda synchronize involved (vram vs ram sync transfers) the numbers can skew if the cards perform differently in that respect. The 3060 might benefit from its higher pcie rating for these transfers. Note that this is somewhat educated guessing and not gospel, but you could try some different nets to see if those compare closer to the 3x to 4x difference. If they do, the m40 is doing fine. Turn back on ecc it's one of the cards perks especially 24gb of it -
This. If i where to buy now, id "maybe" go for these as now these are cheaper here than 3090 were im at, They are designed for scalability (a lot of 3090 designs wont like to be or even fit to be 2x next to each other), and have ECC memory which with large nets and extreme logn training times may be beneficial (if a model suddenly crashes/collapses on itself we can be 100% sure it was a fluke bit in one of the first layers for instance). I also strongly suspect theyll use (much) better rated caps etc since they are designed for sustained load instead of burst peak performance; this is speculation though, perhaps there are some stats on burn-out rate between them by miners or something. So why did i say "maybe"? Cause my ears already start to bleed just by looking at fan blowers (they make sense since ofc they are designed for 2-4 arrays and must eject the heat outside of the case). At the time though 3090s where the economical choice, and compared to previous titan pricing, "dirt cheap" lol
-
Yup, performance tanks on windows. Its mostly the drivers to blame; they are optimized to the max for *nix since thats what datacenters and researchers usually run. They essentially just "make them work on windows" but it has lowest priority and are unoptimzed. When new cards come out usually the drivers are buggy as hell in the first few versions on windows while on nix they are mostly "one time right". Once they are "done" for unix they release, and basically go like "ok, now we have time to look if the windows one didnt just compile but actually works too." Ouch lol, and i thought visual gans where heavy stuff pretty much "max workload" for our poor hardware lol. Yeah tf eco is hard to beat with some stuff; im pretty plain/raw with what i need, and since its pure local research not yet embedded into anything yet i dont need to deploy to anything, im in "works for me" heaven lol. To bring gained knowledge to life in actual appllications is up to others.
-
I never messed with NLP, so not fammiliar with the architectures applied there. Where does the memory load come from i wonder? as the training nets still need to fit in the vram, or perhaps the architecture is so large its chained and swapped in/out just like regular trainig does with the train set data? Its really more the pre/postprocessing and when running concurrent inference tasks that I use above 16gb of normal system mem. During trainkng its like 8-12gb, when using between 2 and 6 datafetch workers for 1024x1024 3channel images. Another thing that comes to mind: I used to need double that, and during training it crept up over time, but that was with tensorflow which is memory leak prone/infested. Moved over to pytorch and never looked back.
-
"I am not at liberty to say specifically" (under NDA). btw interstingly enough, in some cases having multiple slightly less powerfull cards can be beneficial; like two 3080s will outperform lne 3090 by a lot. In periods of normal pricing that can be beneficial (of course youd lose the benefit of being able to run >12gb networks, like 1024 res projected gan (paper from last month) for example, which can gobble up to 19gb. a few tb of nvme is nice, but just mostly if working with larger sets to mamage them and their metadata (to keep sets together with the meta i use jsonfiles alongside the original containing various nets ran inference data, so with a 250k source set and 3 inference runs (which then are used as input to train next net in the chain) kt accumulatetees to 1 million files+. However for train result generated ouput sets (of which there are a lot, for comparison) and training cycle pickles saves each 20kims sata is more than enough. Its will depend on the case and workflows used if nvme is beneficial or not. For trainkng it wont matter at all, just spawn enough load workers, so with 24 thread a hdd could keep up (i think, but wouldnt try lol). Just make sure to go tlc or better and try to stick to at least 1tb preferably 2tb drives as their TBW ratings are usually a lot better. Most of all, its a opem door but still: for machine learning, go linux, save yourself a ton of headaches avoiding windows (less ml optimized drivers and it gobbles to much vram).
-
Hi, Looks pretty good, i have a simmilar configuration as you can see on my profile (3900x 12c 24t, 32gb, pro 980, 3090). And am in the same boat as far as training GANs and traiming vision cnns. Cooling: I personally opted for air cooling, for two main reasons: 1. Reliability 2. During multiday/week training things get hot (esp nvme near and or under gpu); so the inside of the case can use all the "whoos" i can get, location of cpu is a nice center, not just relying on the outer edges fans on a case. Doesnt look as nice though, good air coolers are large blobs... but safety/reliability when running high current stuff for days/weeks was paramount to me. Memory: I doubted between 32 or 64gb mem, opted for 32; this worked out well, am regularly above 16 but never over 24-28 (this is mainly when running inferrence by trained networks over 250k+ image sets, uskng anywhere from 4 to 10 parallel processes). Cpu: 12c 24t same story, found it to be a good sweetspot. More than enough threads to have inputstream workers and some additional processing while still be able to use the shstem concurrent for daily stuff while training. When parallel processing (either preprocessing of learning sets or running inference on larges image sets) it allows enough processes to utilize all 24mb vram of the 3090, wkth a few threads to spare so machine stays snappy. Gpu: 3090, youll love the 24gb, allows training of high res gan architectures, AND try out the trained snapshots at the same time no problem. When running trained networks, can apply parallel processing on the set because most nets can fit a multiple of times in vram. Also looked before at the ML cards like a5000 as werecat says but when i bought 3090 was significantly cheaper here, but these are defknatly good choices as well. Tip: limiting to 250w saves approx 30% in noise/heat but just a few % in compute performance. Dont wear out your fans/caps by blasting power like your trying to get that 2 extra fps. When doing long trains hours accumulate a lot quicker on the card than with office of gaming use. Storage: This is where i fell short initially; didnt consider the size of both my datasets but more so the processing speed of 3090 and i tend to snapsnot pickles and progress previews a lot. Running a few experiments a week can accumulate data quickly depending on the research you do. I ended up adding 2tb more (currently have about 5.5tb in ssd storage, 3tb being nvme), not sure if its myy profile yet but i added a crucial mx500). Case: just anything with easy accesible filters, 24hr/d training causes quick collection of dust in them Hope this helps a bit
-
MSI Suprim 3080 - 3rd fan started to spin full speed
Bartholomew replied to MardyMarvin's topic in Graphics Cards
Thats great! Thanks for reporting back enjoy your rivived card! -
pc build Help me i built a new pc !!
Bartholomew replied to Shivi's topic in New Builds and Planning
Did you try installing windows while the ssd is in the new machine? Sounds like you build a perfectly well working machine but windows just has a hard time adjusting between old and new machine. Often that works, but not always. -
3900x still good in 2021?
Bartholomew replied to frozensun's topic in CPUs, Motherboards, and Memory
I think its not the processor that is the core of the issue here. Somebody fell for "game cache" marketing and bought a production cpu for gaming because of it, at a price that if would have bought a 3600x could now have upgraded to 5600x for free. Lesson learned. -
Nvme ssd won't recognize when Elgato Cap card is installed!?
Bartholomew replied to TheThurston13's topic in Troubleshooting
Unfortunate, but by design if im not mistaken: -
That is a shitton of memory 4 or 2 sticks? Could try 1 stick at a time, for a 1st try. For a 2nd try, More rigorus, but triggered by this: i have a hunch that either some stray conductive dirt or a bad connection is at play. matter of full dissasemble - clean - reassemble then likely does the trick (if you are confident enough of course or build the thing in the 1st place).